Forecasting Business Demand with AI
- Tretyak
- Mar 4, 2024
- 3 min read
Updated: 2 days ago
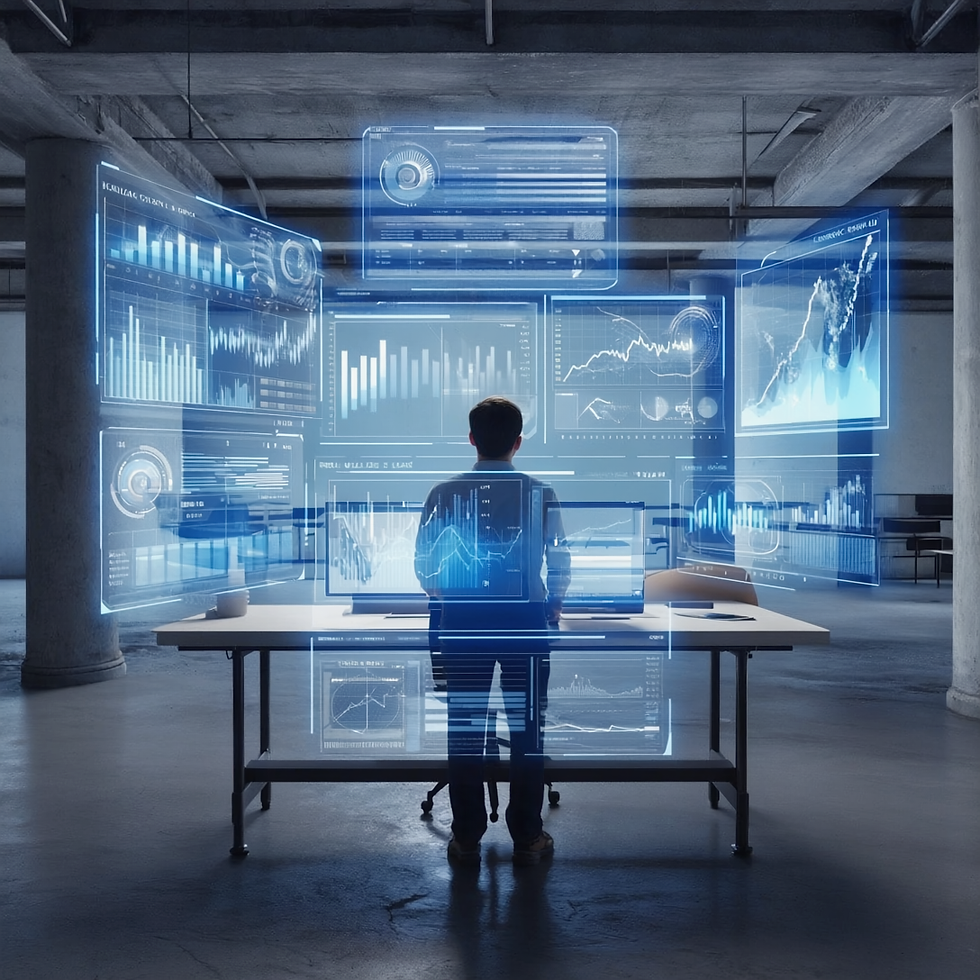
Accurate demand forecasting is the lifeblood of any successful business, enabling optimal resource allocation, inventory management, and strategic planning. Traditionally, this process has relied on historical data and subjective judgment. However, the advent of Artificial Intelligence (AI) has ushered in a new era of precision and insight. Let's delve into the specific mechanisms, applications, and strategic implications of forecasting business demand with AI.
I. Core Mechanisms: AI as a Predictive Engine
Multivariate Time-Series Analysis:
Description: AI algorithms analyze multiple time-dependent variables to identify complex patterns and predict future demand.
Detailed Functionality:
AI models incorporate diverse data sources, including sales data, marketing spend, weather patterns, economic indicators, and social media sentiment.
Advanced statistical techniques, such as ARIMA, SARIMA, and Prophet, are used to model time-series data.
Machine learning algorithms, particularly recurrent neural networks (RNNs) and long short-term memory (LSTM) networks, capture complex temporal dependencies.
Impact: Enables accurate demand predictions by considering a wide range of influencing factors.
Machine Learning-Based Demand Prediction:
Description: AI algorithms learn from historical data and identify patterns that humans might miss, improving forecasting accuracy.
Detailed Functionality:
Regression models (linear, polynomial, support vector regression) predict continuous demand values.
Classification models (logistic regression, decision trees, random forests) predict demand categories (e.g., high, medium, low).
Clustering algorithms (k-means, hierarchical clustering) segment customers and identify demand patterns within specific groups.
Impact: Enhances forecasting accuracy by leveraging the power of machine learning to identify complex relationships in data.
Real-Time Data Integration and Adaptive Forecasting:
Description: AI incorporates real-time data from various sources to adapt forecasts to changing market conditions.
Detailed Functionality:
Real-time data streams from social media, news feeds, and sensor networks are integrated into forecasting models.
AI algorithms continuously monitor and update forecasts based on new information.
Anomaly detection techniques identify unexpected events and adjust forecasts accordingly.
Impact: Enables businesses to respond quickly to market fluctuations and improve forecasting accuracy in dynamic environments.
Personalized Demand Forecasting and Customer Segmentation:
Description: AI segments customers and creates personalized demand forecasts for different customer groups, enabling targeted marketing and inventory management.
Detailed Functionality:
Customer segmentation algorithms (RFM analysis, demographic clustering) identify distinct customer groups.
Personalized demand forecasts are generated for each customer segment based on their specific purchasing behavior and preferences.
Targeted marketing campaigns and promotions are developed based on personalized demand forecasts.
Impact: Optimizes inventory management, improves customer satisfaction, and increases revenue through targeted marketing.
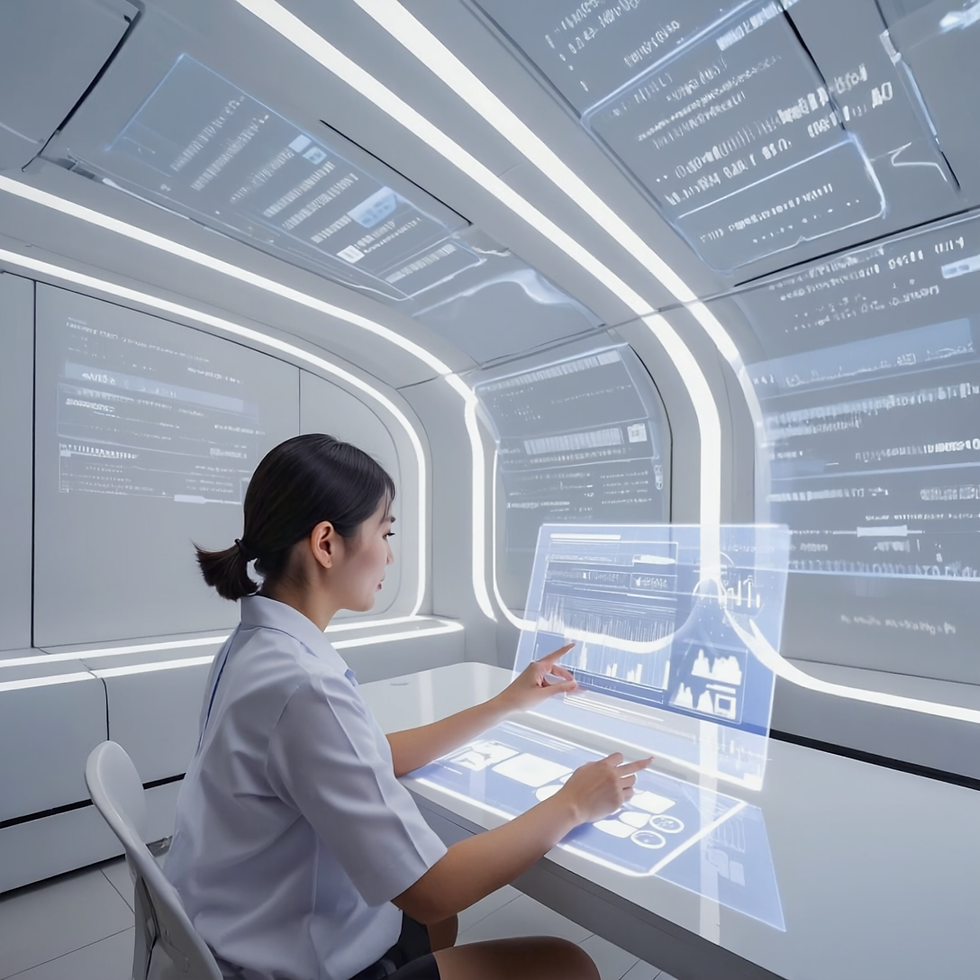
II. Key Technologies and Methodologies:
Time-Series Analysis: Statistical techniques for analyzing time-dependent data.
Machine Learning (ML): Algorithms that learn from data and make predictions.
Natural Language Processing (NLP): Techniques for analyzing text data, such as social media sentiment.
Data Visualization: Tools for presenting forecasting results in an understandable format.
Cloud Computing: Infrastructure for storing and processing large datasets.
III. Strategic Implications and Considerations:
Inventory Optimization: AI-driven forecasts enable businesses to optimize inventory levels, reduce stockouts, and minimize holding costs.
Supply Chain Management: AI enhances supply chain visibility, improves demand planning, and reduces lead times.
Marketing and Sales Strategy: AI informs pricing strategies, targeted promotions, and personalized marketing campaigns.
Resource Allocation: AI enables efficient resource allocation by predicting demand for labor, materials, and equipment.
Risk Management: AI identifies potential risks and uncertainties in demand forecasts, enabling businesses to develop contingency plans.
Ethical Considerations: Addressing potential biases in AI algorithms and ensuring responsible use of forecasting data.
Data Governance: Ensuring data quality, security, and compliance with privacy regulations.
IV. Future Directions:
Integration of Explainable AI (XAI): Making AI forecasting models more transparent and interpretable.
Development of Hybrid Forecasting Models: Combining AI with traditional forecasting techniques.
Real-Time Demand Sensing: Using sensor data and IoT devices to capture real-time demand signals.
Predictive Analytics for New Product Launches: Forecasting demand for new products with limited historical data.
AI-Powered Scenario Planning: Simulating different market scenarios and assessing their impact on demand.
Automated Forecasting Adjustments: AI learning to adjust its own forecasting based on new data and trends.
By harnessing the power of AI, businesses can transform demand forecasting from a reactive to a proactive process, gaining a significant competitive advantage in today's dynamic marketplace.
In conclusion, AI-powered demand forecasting empowers businesses with unparalleled accuracy, adaptability, and the ability to uncover hidden patterns. By leveraging AI, businesses can make data-driven decisions, optimize operations, and gain a competitive edge.

This article highlights the potential of AI for demand forecasting. It's fascinating how it can analyze patterns and improve accuracy compared to traditional methods. I'm curious to learn more about how businesses in my industry can incorporate these AI tools for a competitive advantage.