Improving Diagnostic Accuracy in Healthcare using AI
- Mar 4, 2024
- 3 min read
Updated: May 5
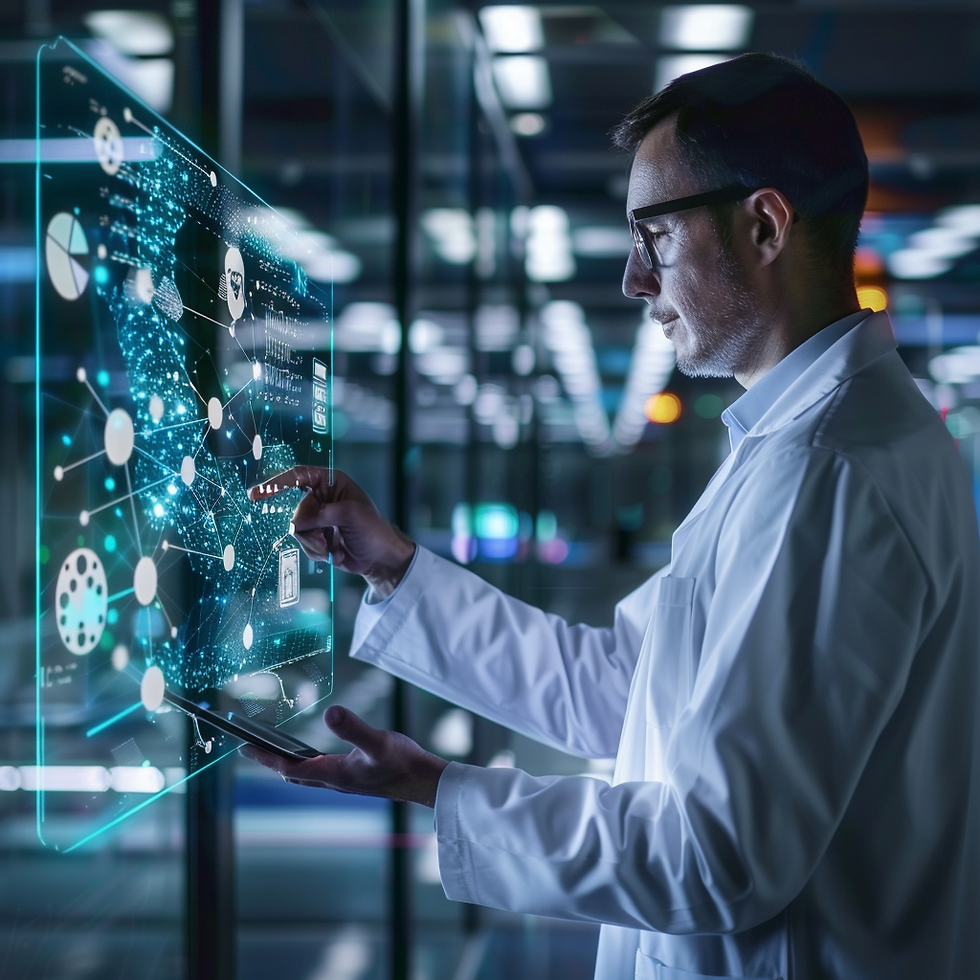
The realm of medical diagnostics is undergoing a profound transformation, propelled by the integration of Artificial Intelligence (AI). Moving beyond traditional methods, AI is becoming a crucial ally for clinicians, enhancing diagnostic accuracy, and ultimately, improving patient outcomes. Let's delve into the specific mechanisms, applications, and ethical considerations of AI in diagnostic healthcare.
I. Core Mechanisms: AI as a Diagnostic Enhancement Tool
Advanced Medical Image Analysis:
Description: AI algorithms analyze medical images (X-rays, MRIs, CT scans, ultrasounds) with exceptional precision, detecting subtle anomalies that might escape human observation.
Detailed Functionality:
Convolutional Neural Networks (CNNs) are employed to identify patterns and features within medical images.
Deep learning models are trained on vast datasets of annotated images to recognize disease-specific characteristics.
AI systems can quantify image features, providing objective and reproducible measurements.
Impact: Enhanced detection of diseases such as cancer, neurological disorders, and cardiovascular conditions, leading to earlier intervention.
Integrated Data Analysis and Pattern Recognition:
Description: AI algorithms integrate and analyze diverse patient data, including medical records, genetic information, laboratory results, and wearable sensor data, to identify complex patterns and correlations.
Detailed Functionality:
Machine learning models, such as support vector machines and random forests, are used to identify relationships between different data points.
Natural Language Processing (NLP) extracts relevant information from unstructured data, such as clinical notes.
AI systems can identify risk factors and predict disease progression based on integrated data analysis.
Impact: Improved accuracy in diagnosing complex diseases, personalized risk assessment, and early detection of disease outbreaks.
Early Disease Detection and Predictive Diagnostics:
Description: AI algorithms can detect early signs of disease, often before symptoms manifest, enabling timely intervention and preventive care.
Detailed Functionality:
AI-powered screening tools analyze patient data to identify individuals at high risk for specific diseases.
Continuous monitoring systems analyze data from wearable sensors to detect subtle changes in physiological parameters.
Predictive models forecast the likelihood of future disease events based on individual risk profiles.
Impact: Earlier detection of diseases such as Alzheimer's disease, diabetes, and cardiovascular disease, leading to improved patient outcomes.
Personalized Diagnostics and Treatment Planning:
Description: AI algorithms can tailor diagnostic approaches and treatment plans to individual patients, considering their unique medical history, genetic makeup, and lifestyle factors.
Detailed Functionality:
AI systems analyze genetic data to identify disease-causing mutations and predict treatment response.
Personalized risk assessment tools tailor screening recommendations based on individual risk profiles.
AI-powered treatment planning systems optimize drug dosages and treatment regimens based on individual patient characteristics.
Impact: Optimized treatment outcomes, reduced adverse effects, and improved patient satisfaction.
Reduction of Diagnostic Errors:
Description: AI algorithms provide objective and data-driven insights, helping to minimize human errors and improve diagnostic accuracy.
Detailed Functionality:
AI-powered decision support systems provide clinicians with evidence-based recommendations.
Automated image analysis tools reduce variability in image interpretation.
AI systems can identify potential drug interactions and contraindications.
Impact: Improved patient safety, reduced medical costs, and enhanced clinical efficiency.
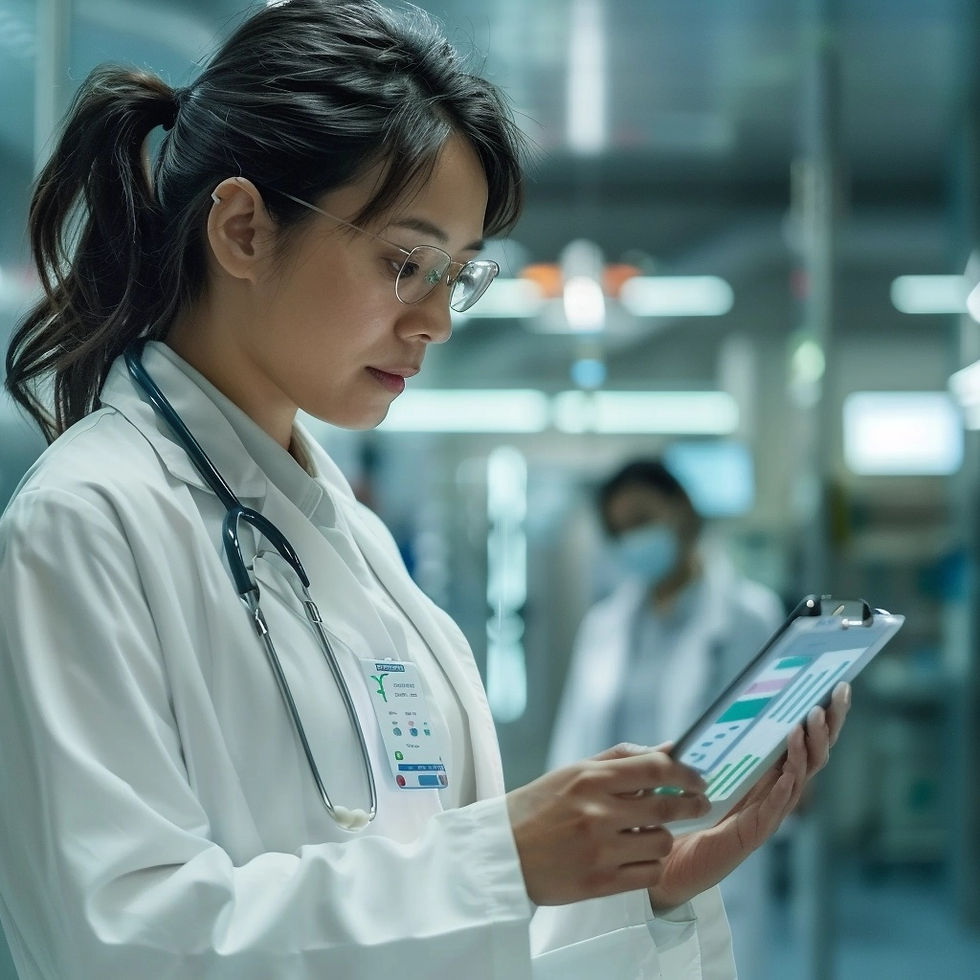
II. Applications Across Medical Specialties:
Radiology: Detection of lung nodules, breast cancer, and stroke.
Pathology: Analysis of tissue samples for cancer diagnosis and classification.
Cardiology: Detection of cardiac arrhythmias, analysis of echocardiograms, and prediction of heart failure.
Dermatology: Diagnosis of skin cancer and other dermatological conditions.
Ophthalmology: Detection of diabetic retinopathy and macular degeneration.
Neurology: Diagnosis of Alzheimer's disease, Parkinson's disease, and multiple sclerosis.
Genetics: Identification of disease-causing mutations and prediction of genetic risk.
III. Ethical Considerations and Challenges:
Data Privacy and Security: Protecting sensitive patient data from unauthorized access and misuse.
Algorithmic Bias: Ensuring fairness and equity in AI algorithms to avoid discriminatory outcomes.
Transparency and Explainability: Making AI models more transparent and understandable to clinicians and patients.
Clinical Validation and Regulatory Approval: Ensuring that AI-powered diagnostic tools are rigorously validated and approved for clinical use.
Human-AI Collaboration: Defining the roles and responsibilities of clinicians and AI systems in the diagnostic process.
Accessibility and Equity: Ensuring that AI-powered diagnostic tools are accessible to all patients, regardless of their socioeconomic status or geographic location.
Liability: Determining liability when an AI makes a incorrect diagnosis.
IV. Future Directions:
Integration of Multimodal Data: Combining data from different sources to create a more comprehensive view of patient health.
Development of Explainable AI (XAI) Models: Making AI models more transparent and interpretable.
Personalized AI Models: Creating AI models that are tailored to the individual patient.
AI-Powered Remote Diagnostics: Enabling remote access to diagnostic services.
AI for Preventative Care: using AI to predict and prevent disease.
By embracing AI responsibly and strategically, we can transform medical diagnostics and improve the lives of patients worldwide.

This is fascinating! It's amazing to see how AI is revolutionizing healthcare. The potential to improve diagnostic accuracy could save countless lives. I'm interested to see how these tools will continue to develop and become more widely adopted in the medical field.