The Algorithmic Arbiters: AI's Dual Role in the Future of Truth and a Resilient Infosphere
- Tretyak
- 2d
- 8 min read
Updated: 12h
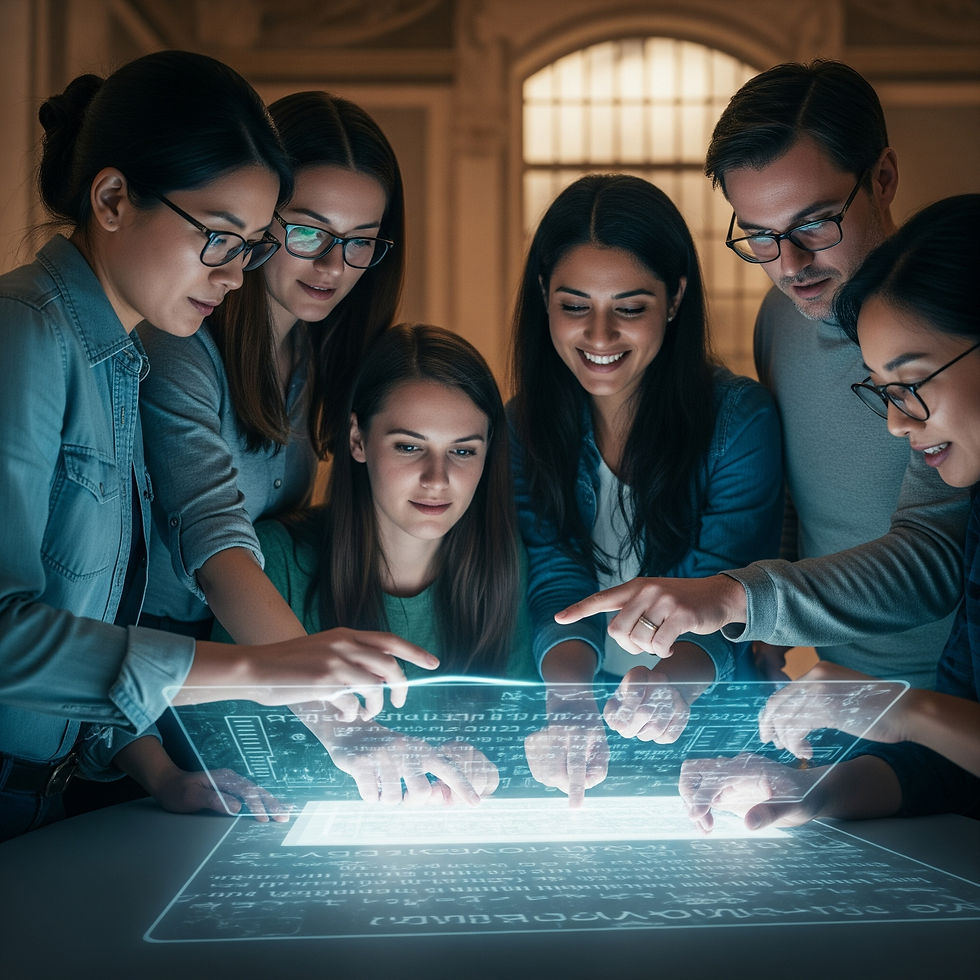
🌐 The Shifting Sands of Shared Reality
Information is the lifeblood of any functioning society, the bedrock upon which collective understanding, democratic discourse, and societal trust are built. Yet, in our hyper-connected digital age, this bedrock is eroding at an alarming rate. The proliferation of misinformation, disinformation, and propaganda—often amplified by the very platforms designed to connect us—has plunged us into a crisis of epistemic trust.
In this turbulent landscape, Artificial Intelligence emerges as a figure of profound paradox: it is simultaneously a powerful engine capable of crafting increasingly sophisticated falsehoods and, potentially, our most crucial ally in defending truth and cultivating a resilient global infosphere.
The stakes could not be higher. The integrity of our democratic processes, the efficacy of public health initiatives, and the very fabric of social cohesion depend on our ability to discern fact from fiction. This post explores AI's complex and dual role—as both a potential forger of deceptive narratives and a discerning detective of inauthenticity. We will delve into the "script" that humanity must collaboratively write to harness AI's capabilities for bolstering truth, mitigating harm, and ensuring that our algorithmic arbiters serve, rather than subvert, a well-informed global citizenry.
🤔 The Double-Edged Algorithm: AI as Prolific Forger and Discerning Detective
AI's impact on the information ecosystem is characterized by a striking duality. Its capabilities can be wielded with equal potency to create deception or to uncover it.
AI as the Architect of Deception:
The evolution of generative AI has equipped those with malicious intent with unprecedented tools. We see this manifest in several ways:
Sophisticated Deepfakes: AI algorithms can create highly realistic but entirely fabricated videos, audio clips, and images, making it difficult for the unaided human eye or ear to detect manipulation.
Persuasive Generative Text: Large Language Models (LLMs) can generate human-quality text at scale, enabling the rapid creation of fake news articles, misleading social media posts, tailored propaganda, and astroturfing campaigns that mimic grassroots support.
Hyper-Personalized Disinformation: AI can be used to tailor deceptive messages to specific individuals or groups based on their psychological profiles and online behavior, increasing the persuasiveness and potential impact of these narratives.
Unprecedented Speed and Scale: AI allows for the creation and dissemination of falsehoods at a velocity and volume that human actors alone could never achieve, overwhelming traditional fact-checking mechanisms.
AI as the Guardian of Authenticity:
Conversely, AI offers powerful capabilities to defend and uphold the integrity of information:
Advanced Digital Forensics: AI tools are being developed to detect the subtle artifacts and inconsistencies present in deepfakes and other forms of manipulated media, acting as a digital fingerprint analysis for the modern age.
Natural Language Processing (NLP) for Detection: NLP algorithms can identify patterns indicative of fake news, analyze the spread of narratives to uncover coordinated inauthentic behavior, detect bot networks, and flag hate speech or harmful content.
Automation in Fact-Checking: AI can assist human fact-checkers by rapidly scanning vast amounts of information, verifying sources, and identifying claims that require further investigation, thereby scaling up fact-checking efforts.
Content Moderation at Scale: For large online platforms, AI provides the only feasible means of reviewing and moderating the immense volume of user-generated content, though this process is fraught with its own challenges.
🔑 Key Takeaways for this section:
AI possesses a dual capacity: it can generate highly convincing fake content (deepfakes, generative text) and also serve as a tool for detecting such manipulations.
AI-driven deception benefits from speed, scale, and personalization, posing significant challenges.
AI-powered detection relies on digital forensics, NLP, and automation to support fact-checking and content moderation.
⚔️ The Current Battlefield: Wins, Losses, and the Evolving Arms Race
The deployment of AI in the infosphere is not a theoretical exercise but an ongoing struggle with tangible consequences. We have witnessed instances where AI tools have been instrumental in identifying and disrupting state-sponsored disinformation campaigns or flagging manipulated media during elections. Social media platforms increasingly rely on AI to detect and remove content that violates their policies, from fake accounts to harmful misinformation.
However, the successes are often shadowed by the relentless advancement of generative AI techniques. The creators of disinformation are constantly refining their methods, leading to an "arms race" where detection tools must continuously adapt to keep pace with new forms of AI-generated falsehoods. Furthermore, so-called "cheap fakes"—less sophisticated manipulations that don't require advanced AI but can still be highly effective—continue to pose a significant problem, sometimes slipping past AI detectors focused on more complex forgeries. The challenge is compounded by the sheer volume of content and the speed at which narratives can propagate across interconnected global networks.
🔑 Key Takeaways for this section:
There's an ongoing "arms race" between AI-powered generation of misinformation and AI-driven detection methods.
While AI has achieved successes in combating disinformation, sophisticated fakes and even simpler manipulations continue to pose threats.
The sheer volume and velocity of information flow challenge even advanced AI detection systems.
🛡️ Beyond Detection: AI's Role in Building Infosphere Resilience
Simply detecting falsehoods after they have spread is often too little, too late. A more proactive and holistic approach involves leveraging AI to build broader resilience within the information ecosystem:
Promoting Media Literacy at Scale: AI-powered educational tools and simulations could help citizens develop critical thinking skills to better identify manipulation techniques, propaganda, and biased reporting on their own.
Enhancing Source Credibility Signals: While challenging to implement without bias, AI could potentially assist in evaluating and signaling the trustworthiness of information sources or individual pieces of content based on various verifiable metrics.
Contextualization and Nuance: Instead of simple "true/false" labels, AI could help provide richer context around disputed claims, offering links to supporting evidence, alternative viewpoints, and explanations of why a piece of information might be contested.
Early Warning Systems: AI algorithms could monitor information flows to identify the early emergence of coordinated disinformation campaigns or potentially harmful narratives before they achieve widespread virality, allowing for quicker countermeasures.
Supporting Quality Journalism: AI tools can assist journalists in research, data analysis, and even in identifying underreported stories, thereby strengthening the sources of reliable information.
The goal is to move beyond a reactive stance to one where AI actively contributes to a healthier, more transparent, and more navigable information environment for everyone.
🔑 Key Takeaways for this section:
AI can play a proactive role in building a resilient infosphere, not just detecting existing falsehoods.
Potential applications include AI-driven media literacy tools, source credibility indicators, contextual information provision, and early warning systems for disinformation.
The aim is to create an environment where citizens are better equipped to navigate information critically.
⚖️ The Algorithmic Judge and Jury: Ethical Quandaries and Governance Imperatives
Entrusting AI with any role in arbitrating or shaping our information landscape brings with it profound ethical dilemmas and an urgent need for robust governance:
The Subjectivity of "Truth": A fundamental challenge is defining "truth," "misinformation," or "harmful content" in a way that can be operationalized by an AI. These concepts are often context-dependent and contested. Who makes these definitions, and whose values do they reflect?
Freedom of Speech vs. Harm Reduction: The use of AI in content moderation inevitably sparks debate about the balance between protecting free expression and preventing the spread of harmful content. Overly aggressive AI moderation can lead to censorship and the suppression of legitimate speech.
Algorithmic Bias: AI systems learn from data, and if that data reflects societal biases, or if the moderation rules are biased, the AI tools can disproportionately flag or suppress content from certain groups, viewpoints, or cultures.
Transparency and Accountability: The decision-making processes of AI content moderation systems are often opaque ("black boxes"). There's a critical need for Explainable AI (XAI) in this domain, as well as clear mechanisms for appeal and redress when errors occur.
The "AI Ministry of Truth" Fear: Concentrating the power to define and enforce truth in the hands of a few entities, whether corporate or governmental, using opaque AI systems, raises dystopian fears of centralized control over information.
Global Governance Complexity: Misinformation is a transnational problem. Developing effective and equitable governance frameworks for AI's role in the infosphere requires international cooperation and sensitivity to diverse cultural and legal contexts.
Navigating these ethical minefields requires careful deliberation, broad stakeholder engagement, and a commitment to upholding fundamental human rights.
🔑 Key Takeaways for this section:
Using AI to arbitrate truth raises critical ethical issues regarding the definition of truth, freedom of speech, algorithmic bias, and accountability.
Transparency (XAI) in AI moderation systems and robust appeal mechanisms are essential.
There are significant risks associated with centralized control over information and the need for global cooperation in governance.
✍️ Forging the "Script" for an AI-Assisted Trustworthy Infosphere
To ensure AI serves as a force for good in our information ecosystem, a concerted and multi-faceted "script" is necessary:
Multi-Stakeholder Collaboration: Tech companies developing AI, social media platforms, governments, academic researchers, media organizations, and civil society must work together to establish norms, best practices, and solutions.
Investment in Research and Development: Continued investment is needed not only in AI for detecting misinformation but also in understanding its societal impact, developing generative AI safety protocols, and creating tools that foster media literacy and critical thinking.
Adaptive Regulatory Frameworks: Governments should consider regulations that promote transparency in AI-generated content (e.g., watermarking or labeling), demand accountability from platforms deploying AI moderation tools, and ensure due process.
Empowering Digital Citizenship: The first and most crucial line of defense is an educated and critical populace. Efforts to promote digital literacy and critical thinking skills must be scaled up, potentially augmented by AI-driven educational tools.
Independent Oversight and Auditing: Establishing independent bodies to audit the AI systems used for content moderation and information curation can help ensure fairness, effectiveness, and accountability.
Prioritizing Context and Plurality: Encourage the development of AI systems that help users access diverse perspectives and understand the context surrounding information, rather than simply making binary true/false judgments.
This "script" must be dynamic, continuously updated to reflect technological advancements and evolving societal understanding.
🔑 Key Takeaways for this section:
A multi-stakeholder approach involving tech, government, academia, and civil society is crucial.
Key actions include R&D investment, adaptive regulation (e.g., labeling AI content), promoting digital citizenship, and ensuring independent oversight of AI systems.
The focus should be on fostering an environment of transparency, accountability, and critical engagement with information.
🏁 Towards Algorithmic Responsibility in the Age of Information
Artificial Intelligence stands as a powerful, ambivalent force in the global infosphere. It offers unprecedented capabilities to both pollute our information ecosystem with sophisticated falsehoods and to cleanse it through advanced detection and resilience-building tools. The path towards a healthier, more trustworthy digital public sphere is not a matter of choosing between AI as a foe or an ally, but of diligently scripting its role as a responsible and accountable partner.
This endeavor requires more than technological fixes; it demands a "whole-of-society" commitment to fostering critical thinking, upholding ethical principles, and designing governance structures that ensure AI augments, rather than undermines, our collective search for truth. The challenge is ongoing, requiring constant vigilance, adaptation, and a global resolve to ensure that our algorithmic arbiters contribute to a future where information empowers, rather than deceives.
How do you believe AI can best be harnessed to combat misinformation while upholding freedom of expression and avoiding bias? What role should individuals, tech companies, and governments play in shaping a trustworthy digital future? Share your thoughts in the comments below.
📖 Glossary of Key Terms
Infosphere: The global environment of information and communication, including digital networks, media, and data.
Misinformation: False or inaccurate information that is spread, regardless of intent to deceive.
Disinformation: False information that is deliberately created and spread to deceive people.
Deepfake: AI-generated synthetic media (video, audio, images) in which a person's likeness is replaced or manipulated to appear authentic.
Generative AI: Artificial intelligence capable of creating novel content, such as text, images, audio, or video.
Large Language Models (LLMs): AI models, typically based on deep learning, trained on vast amounts of text data to understand, generate, and manipulate human language.
Astroturfing: The deceptive practice of creating a false impression of widespread grassroots support for a product, policy, or opinion.
Natural Language Processing (NLP): A subfield of AI focused on enabling computers to process, understand, and generate human language.
Digital Forensics: The practice of collecting, examining, and analyzing digital evidence.
Content Moderation: The process of reviewing and monitoring user-generated content on online platforms to ensure it complies with specific guidelines and policies.
Explainable AI (XAI): Artificial intelligence techniques that aim to make the decisions and outputs of AI systems understandable to humans.
Media Literacy: The ability to access, analyze, evaluate, create, and act using all forms of communication.
Digital Citizenship: The responsible and ethical use of technology, including critical engagement with online information.

Commentaires