The Best AI Tools in Social Sciences
- Tretyak
- Apr 18
- 6 min read
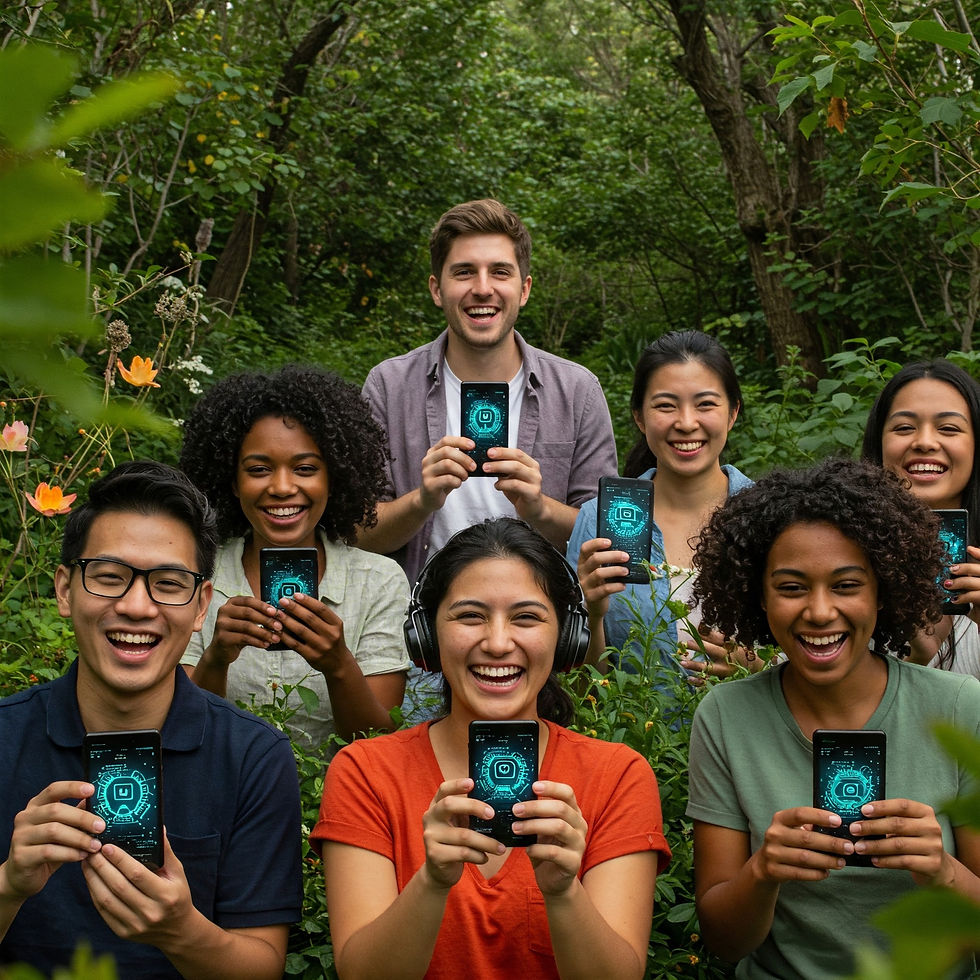
I. Data Analysis & Statistical Modeling
Python Libraries (scikit-learn, pandas, NumPy):
Summary: These are fundamental Python libraries that form the backbone of much data analysis and machine learning in social sciences.
scikit-learn: Provides a wide range of machine learning algorithms for tasks like classification (e.g., predicting group membership), regression (e.g., modeling relationships between variables), clustering (e.g., identifying subgroups), and dimensionality reduction (e.g., simplifying complex data).
pandas: Offers powerful data structures (like DataFrames) for organizing, cleaning, manipulating, and analyzing structured data, which is essential for preparing data for AI/ML algorithms.
NumPy: Provides efficient numerical computing capabilities for working with arrays and matrices, crucial for mathematical operations in statistical modeling and machine learning.
Links:
scikit-learn: https://scikit-learn.org/stable/
pandas: https://pandas.pydata.org/
NumPy: https://numpy.org/
Relevance: Social scientists use these libraries to analyze survey data, demographic information, economic indicators, and other quantitative data to test hypotheses, identify patterns, and build predictive models.
R Statistical Software:
Summary: R is a free and open-source programming language and software environment specifically designed for statistical1 computing and graphics. It's highly extensible, with a vast ecosystem of packages for various statistical techniques and data visualization, making it a favorite in social science research. Many machine learning packages are also available for R.
Relevance: Social scientists use R for statistical analysis, data visualization, and increasingly for machine learning applications like regression modeling, time series analysis, and cluster analysis.
IBM SPSS Statistics:
Summary: SPSS is a commercial statistical software suite that provides a user-friendly interface for performing a wide range of statistical analyses. While traditionally known for its point-and-click interface, SPSS is now incorporating more advanced AI capabilities, such as automated modeling and pattern recognition, to assist researchers in uncovering insights from complex datasets.
Relevance: SPSS is used in various social science disciplines, including psychology, sociology, political science, and economics, for tasks like descriptive statistics, hypothesis testing, regression analysis, and factor analysis.
II. Natural Language Processing (NLP)
Google Cloud Natural Language API:
Summary: This API provides a suite of NLP tools for analyzing text data. Key features include sentiment analysis (determining the emotional tone of text), entity recognition (identifying key people, places, and organizations), text classification (categorizing text into predefined groups), and syntax analysis (understanding the grammatical structure of sentences).
Relevance: Social scientists use this API to analyze textual data from sources like social media posts, news articles, open-ended survey responses, and historical documents. This helps them study public opinion, identify trends, and understand social dynamics.
Amazon Comprehend:
Summary: Similar to the Google Cloud Natural Language API, Amazon Comprehend provides NLP capabilities for tasks like sentiment analysis, entity recognition, topic modeling (identifying key themes in text), and language detection.
Relevance: Researchers use Comprehend to analyze large volumes of text data to extract insights into attitudes, opinions, and social phenomena.
Hugging Face Transformers:
Summary: This is an open-source library that provides access to pre-trained transformer models, such as BERT, GPT, and RoBERTa. These models are highly advanced NLP systems that can perform a wide range of tasks, including text classification, question answering, text generation, and language translation.
Relevance: Transformers are used in social sciences for sophisticated text analysis, such as understanding complex language patterns, identifying misinformation, and generating realistic text for simulations.
III. Social Media Analysis
(Social Media Analytics Platforms with AI):
Summary: These platforms use AI to analyze social media data to understand trends, track sentiment, identify influencers, and map social networks.
Sprout Social: A platform for social media management and engagement, with AI features for sentiment analysis and reporting.
Brandwatch (Meltwater): A social listening platform that uses AI to analyze online conversations and track brand mentions.
Links:
Sprout Social: https://sproutsocial.com/
Brandwatch (Meltwater): https://www.meltwater.com/en
Relevance: Social scientists use these tools to study public opinion, social movements, and the spread of information on social media.
IV. Survey & Questionnaire Analysis
Qualtrics:
Summary: Qualtrics is a powerful survey platform that is incorporating AI to enhance survey design, distribution, and analysis. AI features include automated data cleaning, sentiment analysis of open-ended responses, and predictive analytics to identify key drivers of satisfaction.
Relevance: Survey research is a core methodology in many social sciences, and Qualtrics' AI tools can help researchers collect and analyze survey data more efficiently and gain deeper insights.
V. Agent-Based Modeling
NetLogo:
Summary: NetLogo is a programmable modeling environment for agent-based modeling (ABM). ABM is a computational approach that simulates the actions and interactions of autonomous agents (e.g., individuals, organizations) to understand complex social systems. While NetLogo itself is not an AI tool, it can be used to build simulations that incorporate AI elements, such as agents that learn and adapt.
Relevance: Social scientists use ABM to study phenomena like the spread of diseases, the dynamics of social networks, and the behavior of markets.
VI. Network Analysis
Gephi:
Summary: Gephi is an open-source software for network analysis and visualization. It helps researchers to visualize and analyze relationships between individuals, groups, or entities in a network. While Gephi is primarily a visualization tool, AI techniques can be applied to enhance network analysis, such as using machine learning to identify communities or predict network evolution.
Link: https://gephi.org/
Relevance: Social network analysis is a key method in sociology, communication studies, and political science, and Gephi provides a powerful tool for understanding social structures and interactions.
VII. AI Development Platforms (General Use, but Applicable)
Google Cloud Vertex AI:
Summary: Google Cloud's Vertex AI provides a platform for building and deploying custom machine learning models. Social scientists can use Vertex AI to develop models tailored to their specific research questions, such as predicting social trends or classifying text data.
Relevance: Offers a scalable and powerful environment for developing and experimenting with AI models.
Amazon SageMaker:
Summary: Similar to Vertex AI, Amazon SageMaker is an AWS platform for building, training, and deploying machine learning models. Social scientists can use SageMaker for tasks like building predictive models or analyzing large datasets.
Relevance: Provides a comprehensive set of tools and services for machine learning development.
Microsoft Azure Machine Learning:
Summary: Microsoft Azure Machine Learning is another cloud-based platform for building and deploying AI models. Social scientists can use Azure ML for tasks like building classification models or performing time series analysis.
Link: https://azure.microsoft.com/en-us/services/machine-learning/
Relevance: Offers a cloud environment with various machine learning services and tools.
VIII. Research-Focused AI Tools & Libraries
spaCy:
Summary: spaCy is a Python library for advanced natural language processing. It provides tools for tokenization, part-of-speech tagging, named entity recognition, and dependency parsing, which are useful for analyzing the structure and meaning of text.
Link: https://spacy.io/
Relevance: Researchers in fields like linguistics, communication studies, and sociology use spaCy to analyze text data and extract linguistic features.
NLTK (Natural Language Toolkit):
Summary: NLTK is another Python library for NLP, offering a wide range of tools for text processing, such as tokenization, stemming, and sentiment analysis. While spaCy is known for its speed and efficiency, NLTK provides a broader range of functionalities.
Link: https://www.nltk.org/
Relevance: Similar to spaCy, NLTK is used for analyzing text data in social science research.
NetworkX:
Summary: NetworkX is a Python library for creating, manipulating, and analyzing the structure and dynamics of networks. It provides tools for calculating network measures, such as centrality and clustering, and for modeling network evolution.
Link: https://networkx.org/
Relevance: NetworkX is used in sociology, political science, and communication studies to study social networks, organizational structures, and information flows.
Statsmodels:
Summary: Statsmodels is a Python module that provides classes and functions for estimating various statistical models, conducting statistical tests, and exploring statistical data. While not strictly an AI tool, it's essential for statistical analysis that often precedes or accompanies AI/ML applications.
Relevance: Used to build and evaluate statistical models that form the basis for some AI predictions.
IX. Qualitative Data Analysis Software (with emerging AI)
NVivo:
Summary: NVivo is a software package for qualitative data analysis. It helps researchers organize, code, and analyze non-numerical data like interview transcripts, field notes, and documents. While traditionally a qualitative tool, NVivo is starting to incorporate AI features to assist with tasks like automated coding and theme extraction.
Link: https://www.qsrinternational.com/nvivo-qualitative-data-analysis-software/
Relevance: Qualitative research is crucial in disciplines like sociology, anthropology, and psychology, and AI is beginning to augment these methods.
X. AI for Agent-Based Modeling
Mesa:
Summary: Mesa is a Python framework for building and running agent-based models (ABM). ABM simulates the behavior and interactions of individual agents to understand how they collectively influence the system. While Mesa itself is a modeling tool, researchers can incorporate AI into the agents' decision-making processes.
Relevance: ABM is used to study complex social phenomena, such as the spread of information, the dynamics of markets, and the evolution of social norms.
AnyLogic:
Summary: AnyLogic is a simulation software that supports agent-based, discrete event, and system dynamics modeling. Similar to Mesa, AnyLogic can be used to build simulations that include AI-driven agents.
Relevance: Provides a versatile platform for simulating complex systems, including those relevant to urban planning, economics, and organizational behavior.
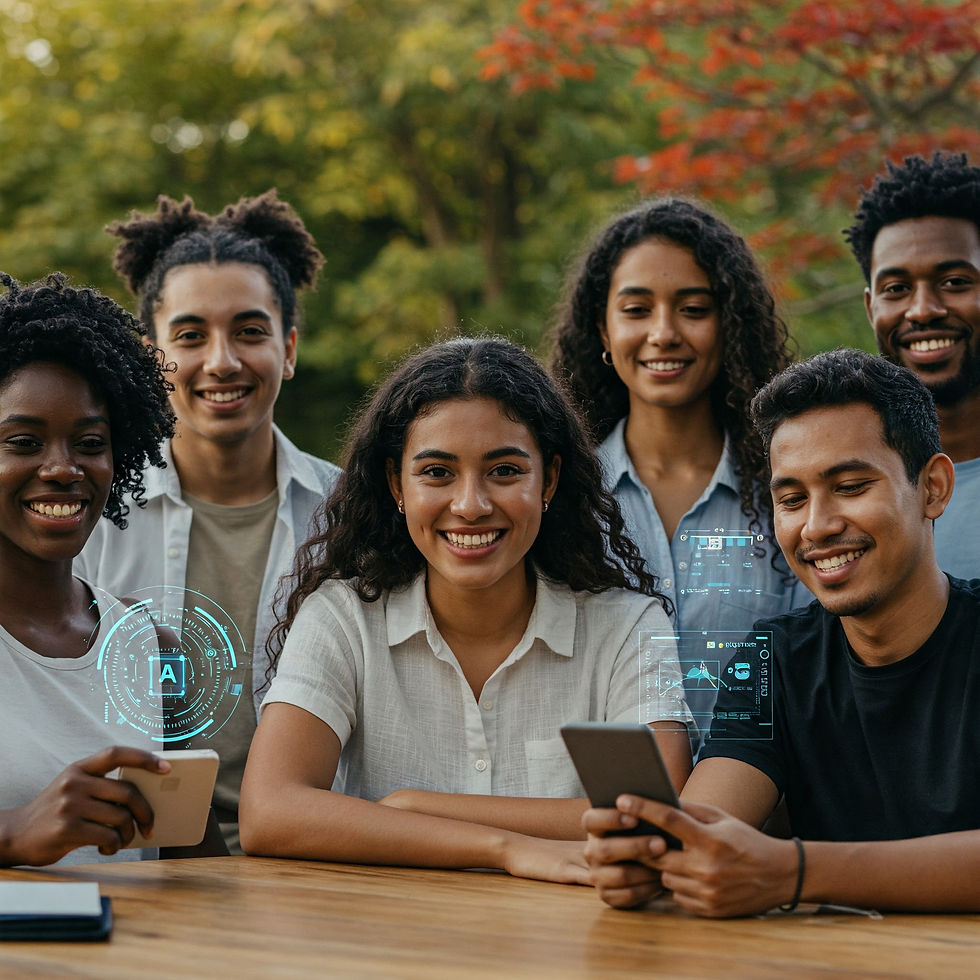
Comentarios